
- #CHECK NVIDIA CUDA TOOLKIT VERSION HOW TO#
- #CHECK NVIDIA CUDA TOOLKIT VERSION INSTALL#
- #CHECK NVIDIA CUDA TOOLKIT VERSION DRIVER#
To check which version of CUDA and CUDNN is supported by the hardware or the GPU that is installed in your computer.
#CHECK NVIDIA CUDA TOOLKIT VERSION DRIVER#
This suggests again that cuda is not included in the display driver installation.How to check Cuda Version compatible with installed GPU comments: "will display CUDA Version even when no CUDA is installed." It does not show if Cuda is actually installed. The answer of using nvidia-smi to get the version in the top right is rejected as wrong since it only shows which version is supported.
#CHECK NVIDIA CUDA TOOLKIT VERSION INSTALL#
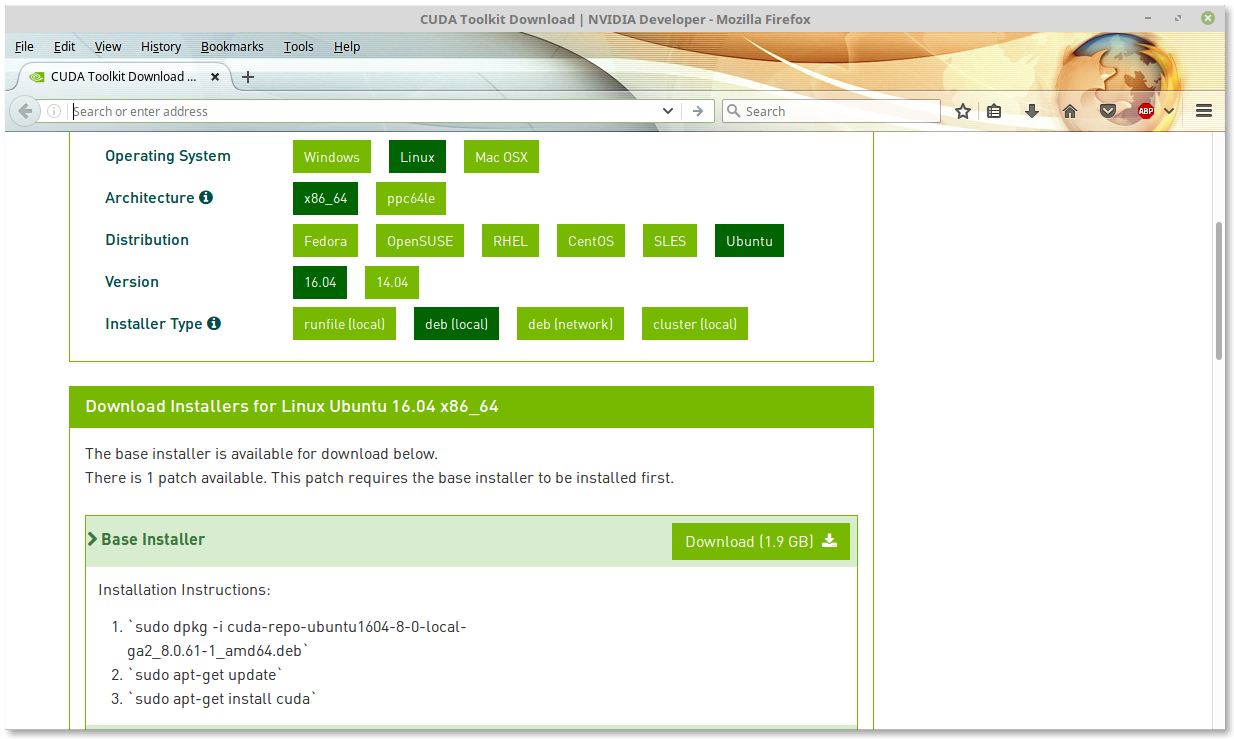
The installation then installs a cuda toolkit: It would not install cuda if that came with the display driver. Going to, you get conda install pytorch torchvision cudatoolkit=10.2 -c pytorch as the installation command in conda prompt. Why not just testing an installation that needs cuda to find out. Thus: try conda, and only if that does not work, try pip. UnsatisfiableError: Finds incompatible specification for CUDA driver, even though I have the version in the specification, and pip installs fine - installing PyMC3 and tensorflow. Conda instead is recommended by NVIDIA since it handles the dependencies.ĮDITED: I found an example of what was also commented: that pip sometimes works when conda does not. The reason: in both pip installs (1./2.), there is no guarantee that your dependencies work in all settings. With pip, you can use "CUDA Toolkit" (1.), but you should not! You can also install "cudatoolkit" (2.) with pip, but that is also not recommended. When using anaconda installer ( conda install tensorflow-gpu), you do not need to install the system "CUDA Toolkit" (standalone, meaning outside of Python). EDIT: Please mind that using Anaconda to install tensorflow is recommended, see and a guide at.

Pytorch from source (if you have an older graphics card and you need to build your own pytorch version with all dependencies): with standalone / system CUDA Toolkit and standalone / system cuDNN before you install pytorch, see and a guide at.
#CHECK NVIDIA CUDA TOOLKIT VERSION HOW TO#
You can get this done with pip, but setting it up like this is for example not recommended by pytorch who recommend conda: "Anaconda is our recommended package manager since it installs all dependencies." And since conda cannot use the "CUDA Toolkit", see How to run pytorch with NVIDIA "cuda toolkit" version instead of the official conda "cudatoolkit" version?, using "CUDA Toolkit" is not recommended either, which should mean the same for Tensorflow - and it does, see the last bullet point. Standalone / system CUDA Toolkit: with executable install: with called "CUDA Toolkit" if you need it for other purposes outside of anaconda.You do not need the system "CUDA Toolkit", see at. At the moment, "cudatoolkit" with maximum version 10.2 can be chosen as the conda install parameter. It is not recommended to install "cudatoolkit" and cudnn manually, use conda install command with all its dependencies. In the following, "CUDA Toolkit" (standalone, what you would install outside of Python right on your system) and cudatoolkit (conda) are different! The conda install command for Pytorch will need the conda install parameter "cudatoolkit", while tensorflow does not need the parameter. Tensorflow and Pytorch do not need the CUDA system install if you use conda (recommended).Tensorflow and Pytorch need the CUDA system install if you install them with pip without cudatoolkit or from source.
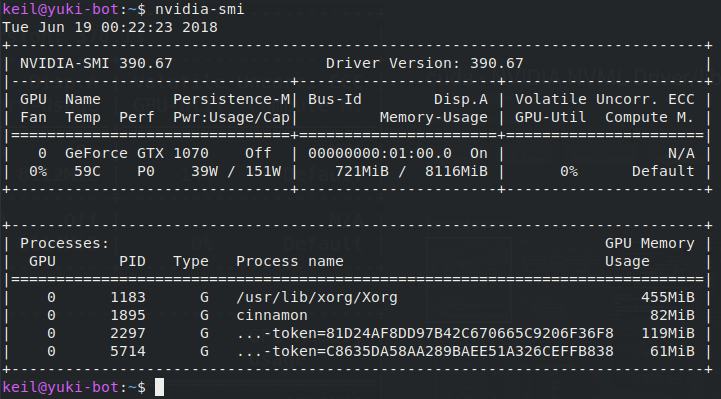
